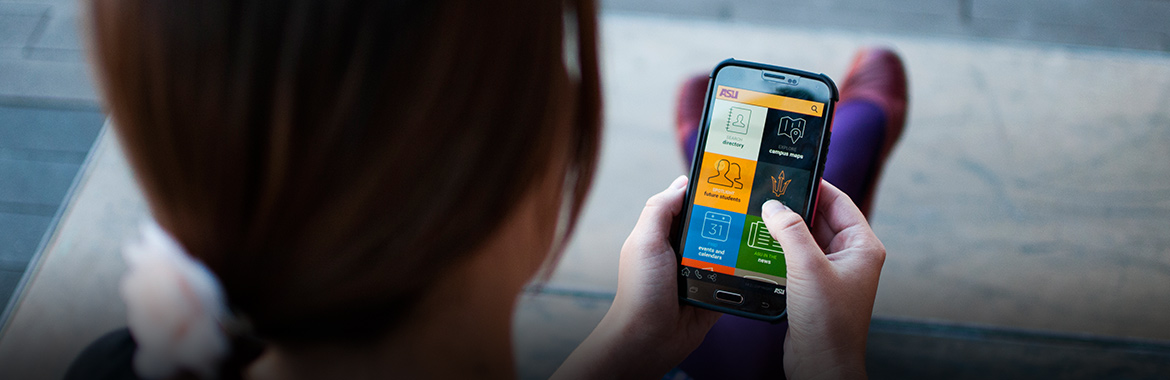
In The News
Lessons in Experimentation
We as consumers witness experiments daily, most without realizing. If you’ve ever received an email seemingly out-of-the blue with a 15% off coupon to a retailer that you haven’t visited in a while, you’ve likely been part of an experiment. Whether or not we jump at the opportunity provides data to the company on if the experiment was successful and if not, what could be the cause. “You can’t shake a stick online without hitting an experiment,” Anson Park and Edgar Hassler mention.
Anson and Edgar conduct an array of online experiments for American Express. “OCEAn is an acronym we hope becomes popular for Online Controlled Experimentation and Analysis,” Anson explains. We’ve seen other names and acronyms like OCE, COE, and “Continuous Experimentation”, and none of them seem like they’d lend themselves to a good t-shirt design. OCEAn, on the other hand, would lend itself to excellent t-shirt designs.”
Both Edgar and Anson are former students of Dr. Doug Montgomery, a regents professor of industrial engineering and statistics in the Ira A. Fulton Schools of Engineering at ASU, and a well-known expert in the field of experimental design. Their careers revolve around working with engineers and product developers to make digital experiments more efficient and end results more sound.
Anson and Edgar sat down with Dr. Montgomery during his monthly Design of Experiments Fireside Chat with participants of the Design of Experiments Specialization offered through Coursera. With their intentionally-coordinated Hawaiian shirts, Anson and Edgar further discussed the concepts and actions of online experimentation.
Anson and Edgar wanted to emphasize that they are speaking for themselves and not in any official capacity for American Express.
“There’s often a need for experimental design expertise in particular. When you take a statistics course, especially a required introductory course for a non-statistics discipline, you mainly spend time on how to fit a model and/or conduct a test, and very little time on how to set up things to get the best model or most sensitive test. Planning can require domain-specific understanding and is usually an order of magnitude more difficult than the analysis, so it doesn’t get a lot of time,” Hassler and Park explain. “Knowing how to do it well lets you make valuable contributions in a lot of places.”
Anson and Edgar spend a lot of time on the operations side of an experiment working with shareholders in different areas of responsibility with a goal of getting everyone on the same page.
“For any size organization, injecting experimentation into your technology stack can be technically tricky and different methods have different trade-offs. For example, many vendors provide javascript frameworks that take the content of the page and modify it according to the experimental parameters. But this causes a negative side effect known as `flicker`, where the user may notice elements of the webpage vanishing and reappearing but now somehow different. Some vendors offer server-side frameworks to render the experiment before it’s sent to the user, but this can cause issues with content distribution networks. When an experiment can touch any place in your technology stack, then everyone responsible for any of those touchpoints has to be brought along.”
One of the more challenging parts of experimentation is convincing people to test frequently and to be bold with their ideas. “Often, we talk to people that view a negative result as a failed test,” Edgar explains. “That’s really a successful test. You’ve learned something. A failed test is one that tells you something that isn’t true.”
There’s a number of ways for a test to tell you something that isn’t true. These are the traditional type 1 and type 2 errors in hypothesis testing. However, Anson and Edgar noted that this is not the whole picture.
“Let’s start with how inferential errors for standard A/B tests are normally presented in textbooks. If the null hypothesis is true and no effect is present then rejecting the null hypothesis is a type 1 error. If some alternative hypothesis is true and we fail to reject the null hypothesis—which is no longer true in this case, then a type 2 error has occurred.”
“If we did a two-sided test then an effect being negative but looking positive is not technically an error. We successfully identified that the null was not true, but in practice this is the most serious kind of error. Now, let’s say instead we did a one-sided test, then a negative effect doesn’t even enter the picture since it is neither the null hypothesis nor is it one of the alternatives. Usually we ignore these negative-effect-appears-positive because they are much less likely to occur than the type 1 errors themselves, and historically people have been very concerned with type 1 error rates.”
“This primacy of the type 1 error rate in planning occurs in lots of places, and for good reason. In industrial and agricultural settings, committing a type 1 error means we have wasted a bunch of time and money retooling, replanting, and retraining. In medical settings, it can mean a person underwent a painful procedure for no reason, or that they passed on a truly effective procedure in favor of one that does nothing. The true cost of a type 1 error in both settings is a major concern.”
However, a type 1 error in an online controlled experiment is often not very serious. “The result of these kinds of experiments just tells us which branching path of our code to keep and we’ll delete the rest. We’ve already spent the money to develop the different variants, so that cost is sunk.” In this case, a type 1 error would produce almost no real impact to the business. The real impact would be from negative effects disguised as positive. “As the type 1 error rate increases, so do the rates of these kinds of events.”
As a general rule of practice, Anson and Edgar make a point to consult with colleagues to have them think through the impact errors in inference could have on their bottom line. “We ask them to rethink the knee-jerk instinct to ‘alpha equal to point zero-five and beta equal to point-one.’”
Once an experiment has been conducted, it’s time to sort through the data and analyze the results. “The most important thing we need to do is track which users were exposed to which experimental variants, and determine what they did.” This can be very challenging in settings where a user doesn’t immediately log in to an account or uniquely identify themselves by some other means.
“These users may start browsing on their phone, then switch to their desktop. They may decide to come back over the weekend on a tablet. Beyond these inherent difficulties, there may be legal roadblocks as well. They may have privacy requirements that prohibit us from tracking them. We’re all still trying to figure out the best way to handle these situations.”
However, Anson and Edgar say that the difficulties and resource requirements are worth the benefit of online controlled experimentation. The only way to confidently ensure key business metrics are moving in the right direction is through performing controlled online experimentation with statistically valid analysis. “No amount of good practice will make a pool of bad ideas into good ones, but if there are some good ideas in that pool then rigorous methods will help you get to them.”
The future of online experimentation continues to shine. “It’s hard to defend not being a data driven organization anymore. We’re almost 20 years past Moneyball; it’s in the popular culture. If you’re not looking at your data and making smart decisions based on that then someone else will, and they will eat your lunch.” Anson and Edgar noted that having well-thought-out experiments is a crucial part of being a data-driven organization. “Like Dr. Montgomery says, every experiment is a planned experiment, some are just planned poorly.”
“That said, there’s a lot of third parties that push questionable methods. And it’s a hard sell to convince companies to re-run experiments or maintain holdout sets to verify estimates and error rates. It will only get easier to be taken advantage of or to pay lip-service to the idea of being a data driven organization. That’s a danger going forward.”
In a data-rich world, Anson and Edgar find it unfathomable to make decisions without using proper experimentation. Consider that next time you’re browsing a retailer or checking on a bank account online. What experiment are you actively taking part in and what are you telling the company?
The full recording of Anson and Edgar’s Fireside Chat can be found here. To learn more about the Design of Experiments Specialization offered through Coursera, please visit ASU’s Global Outreach and Extended Education website.
--
Co-written by Edgar Hassler and Anson Park, American Express, and Meghan Gibson, ASU.
November 2020